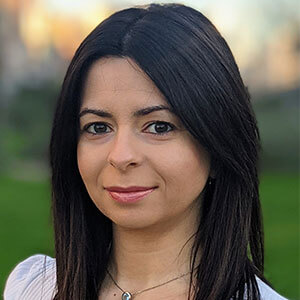
TZOMPANAKI Katerina
Member of team :
MIDI
Phone :
01 34 25 66 28
Biography
Since September 2016, Katerina Tzompanaki is an associate professor (Maitre de Conferences) at the CY Cergy Paris University and a member of the MIDI team at ETIS lab.
Previously, she did a post-doc in the DbWeb team of Telecom ParisTech, working with Fabian Suchanek on information extraction.
She defended her doctoral thesis with title “Query debugging and fixing to recover missing query results” (pdf) in December 2015. She conducted her PhD research at the University Paris-Sud XI and at the laboratory LRI, in the LaHDAK team. Her PhD supervisors were Nicole Bidoit and Melanie Herschel. She was also member of the OAK Project of INRIA Saclay.
She finished with honours her master studies on Information Systems, namely on the Semantic Web, in the Computer Science Department (CSD) of the University of Crete (UOC) and the Information Systems Laboratory (ISL) of FORTH-ICS under the supervision of Martin Doerr. Before that, she have also worked as an engineer at the same laboratory. She received her Electrical and Computer Science Engineering diploma from the School of Electrical and Computer Engineering, of the National Technical University of Athens (ECE-NTUA).
Katerina Tzompanaki serves as the responsible for the Egality and Parity missions at ETIS lab. She is also the co-responsible of the Master 1 and the Professional Master 2 Programm IISC at CY Cergy Paris. She has been serving as a program member for various conferences in the wider area of databases and artificial intelligence like ICDE, CIKM, IJCAI, VLDB, EDBT and others. She has published her work on data provenance, data privacy, explainable recommenders and semantic web in many top-rank venues like CIKM, VLDB, EDBT, PKDD among others.
Research activities
- Data Provenance
- Data Explainability
- Explainable Machine Learning
- Data Privacy
Ongoing projects
- Data Privacy in Sequential Data (2016-2021)
- Why-Not explanations for Recommender Systems (2020-2023)