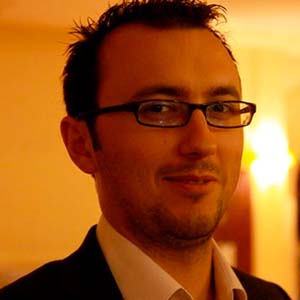
GROZAVU Nistor
Member of team :
MIDI
Phone :
01 34 25 00 00
Biography
Nistor Grozavu received his HdR degree from Sorbonne Paris Nord University in 2020 and PhD degree in Unsupervised Machine Learning in 2009 from Paris 13 University.
He is currently Full Professor in Computer Science at CY Cergy Paris University. His research is with the MIDI team from ETIS Laboratory. His research interests include Unsupervised Learning, Transfer Learning, Dimensionality reduction, Collaborative Learning, Machine Learning by Matrix Factorization and content-based information retrieval, Quantum Machine Learning. These researches are applied in different applications for text mining, visual information retrieval, recommendation systems, fraud detection, etc. via ANR, FUI, PEPS CNRS, AUF projects. He is also a member of IEEE, INNS, and the co-founder of the INNS Autonomous Machine Learning group. Nistor is the co-author of a patent on visual information retrieval, published two book chapters, 12 peer-reviewed journal papers, and more than 40 international conference papers. Nistor Grozavu co-supervised 2 post-doctorants, 8 PhD students and supervise each year 1-2 Master2 internship.
Research activities
- Multimodal Machine Learning
- Unsupervised Machine Learning
- Topological Clustering
- Multi-view clustering
- Representations learning
- Dimensionality reduction
- Learning Data Streams
- Interpretability in Unsupervised Machine Learning
- Opinion mining
- Recommendation systems
- Visual information retrieval
Ongoing projects
- ANR Pro-TEXT (2019-2024) – Local responsible
Pro-TEXT (Processes of Textualization: Linguistic, Psycholinguistic, and Machine Learning Modeling) is an innovative interdisciplinary project putting under scrutiny
a new research object, the dynamics of the textualisation process. The aim is to elucidate the way a complex and globally constrained unit, the text, is built out of
simple and locally constrained linguistic units. - Industrial project with Synaltic (2019-2022) – co-responsible for the project
The goal of the project is to use unsupervised machine learning at the service of data quality - Industrial project with Fi Group (2021-2024) – responsible for the project
The aim of the project is to learn the representations of large text streams data using unsupervised machine learning - Industrial project with ALTECA (2021-2024) – responsible for the project
Create an Emotional Response Recommendation for context-aware affective systems based on multi-modal data (text, audio, images).