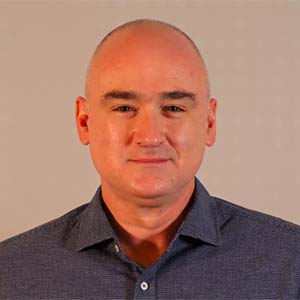
KOTZINOS Dimitrios
Phone :
+33 13425 2855
Biography
Dimitris Kotzinos is a Professor at the Department of Computer Science of the CY Cergy Paris University, member of the ETIS Lab and member of the MIDI team of the lab. He holds a Ph.D. in Computer Science (in Real-Time Web Information Systems) (2001) and a M.Sc. in Transportation (1996), where he studied networks and their applications in transportation systems. His main research interests include data management algorithms, techniques and tools; development of methodologies, algorithms and tools for web-based information systems, portals and web services; and the understanding of the meaning (semantics) of interoperable data and services on the web and also the understanding and summarization of large Knowledge Graphs (KGs). He is working on studying the formation and evolution of discussions in online social networks using Machine Learning (ML) and Artificial Intelligence (AI) techniques, as well as the evolution of social graphs. Additionally, he is also working in the area of accountability, explainability and fairness of the ML and AI algorithms, especially when applied in data engineering and analysis problems; this includes issues on data privacy and especially their intersection with the publication of Linked Open Data. This work extends also in the area of personal mobility and personal social networks. Dimitris has published more than 70 articles in various journals, books, conferences and workshops and serves as a program committee member and reviewer for various conferences and journals. He is also participating in nationally and internationally funded research programs around data analytics, data models and networks and their integration in the everyday life.
Personal web page: www.kotzinos.eu
Research activities
- Data science
- Data analysis
- Graph learning algorithms
- Graph neural networks
- Social media analysis
- Graph prediction based on graph structure
- (Analysis of) personal mobility
- Smart cities
- Data privacy
- Learning-based query optimization
- Learning weak signals in big datasets
- Transparency in AI
- Explainability and fairness in AI
- Enhancing AI fairness through impact assessment in the European Union: a legal and computer science perspective
- Extracting Unique Discussions of Interests for Entrepreneurs and Managers in a Set of Business Tweets Without Any Human Bias
- 3D Modelling Approach for Ancient Floor Plans' Quick Browsing
- Landmark Privacy: Configurable Differential Privacy Protection for Time Series